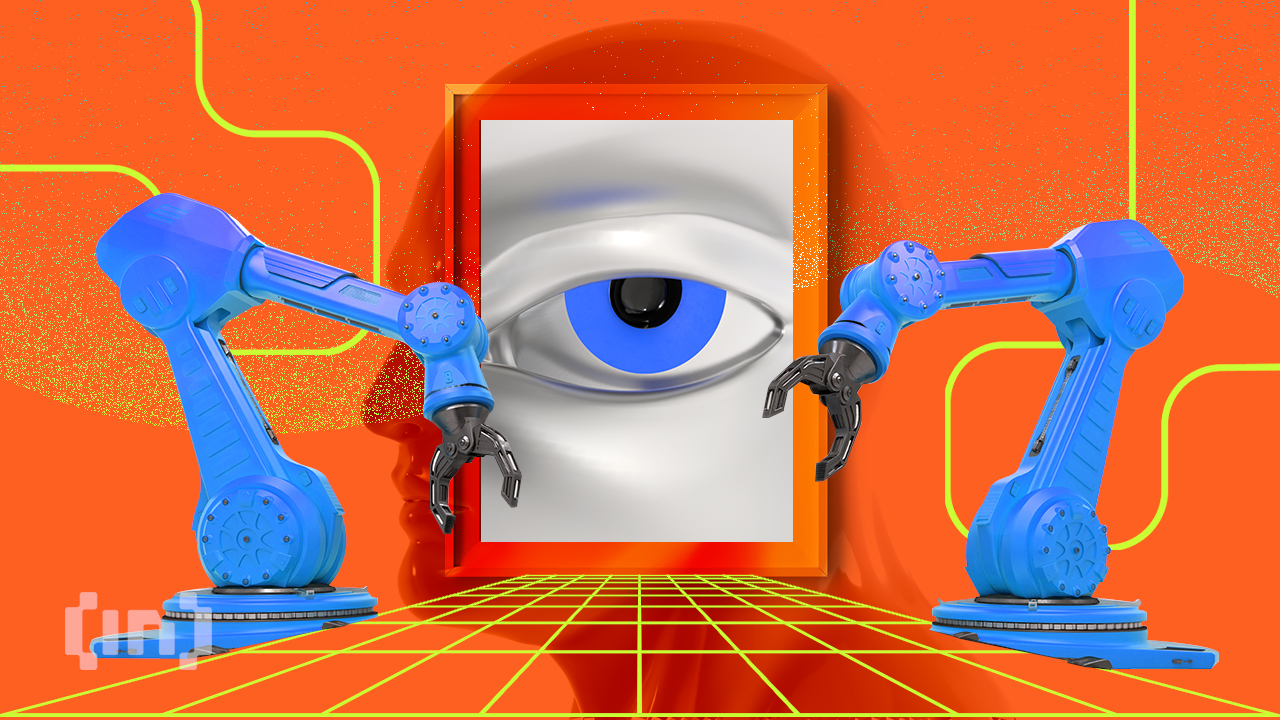
Artificial intelligence’s sophisticated advancements have given rise to Large Language Models (LLMs) such as ChatGPT and Google’s Bard. These entities can generate content so human-like that it challenges the conception of authenticity.
As educators and content creators rally to highlight the potential misuse of LLMs, from cheating to deceit, AI-detection software claims to have the antidote. But just how reliable are these software solutions?
Unreliable AI Detection Software
To many, AI detection tools offer a glimpse of hope against the erosion of truth. They promise to identify the artifice, preserving the sanctity of human creativity.
However, computer scientists at the University of Maryland put this claim to the test in their quest for veracity. The results? A sobering wake-up call for the industry.
Soheil Feizi, an assistant professor at UMD, revealed the vulnerabilities of these AI detectors, stating they are unreliable in practical scenarios. Simply paraphrasing LLM-generated content can often deceive detection techniques used by Check For AI, Compilatio, Content at Scale, Crossplag, DetectGPT, Go Winston, and GPT Zero, to name a few.
“The accuracy of even the best detector we have drops from 100% to the randomness of a coin flip. If we simply paraphrase something that was generated by an LLM, we can often outwit a range of detecting techniques,” Feizi said.
AI-Related Issues. Source: Statista
This realization, Feizi argues, underscores the unreliable dichotomy of type I errors, where human text is incorrectly flagged as AI-generated, and type II errors, when AI content manages to slip through the net undetected.
One notable instance made headlines when AI detection software mistakenly classified the United States Constitution as AI-generated. Errors of such magnitude are not just technical hitches but potentially damage reputations, leading to serious socio-ethical implications.
Read more: UN Report Highlights Dangers of Political Disinformation Caused by Rise of Artificial Intelligence
Feizi further illuminates the predicament, suggesting that distinguishing between human and AI-generated content may soon be challenging due to the evolution of LLMs.
“Theoretically, you can never reliably say that this sentence was written by a human or some kind of AI because the distribution between the two types of content is so close to each other. It’s especially true when you think about how sophisticated LLMs and LLM-attackers like paraphrasers or spoofing are becoming,” Feizi said.
Spotting Unique Human Elements
Yet, as with any scientific discourse, there exists a counter-narrative. UMD Assistant Professor of Computer Science Furong Huang holds a sunnier perspective.
She postulates that with ample data signifying what constitutes human content, differentiating between the two might still be attainable. As LLMs hone their imitation by feeding on vast textual repositories, Huang believes detection tools can evolve if given access to more extensive learning samples.
Huang’s team also zeroes in on a unique human element that may be the saving grace. The innate diversity within human behavior, encompassing unique grammatical quirks and word choices, might be the key.
“It’ll be like a constant arms race between generative AI and detectors. But we hope that this dynamic relationship actually improves how we approach creating both the generative LLMs and their detectors in the first place,” Huang said.
The debate around the effectiveness of AI detection is just one facet of the broader AI debate. Feizi and Huang concur that outright banning tools like ChatGPT is not the solution. These LLMs hold immense potential for sectors like education.
Read more: New Study Reveals ChatGPT Is Getting Dumber
Instead of striving for an improbable, 100% foolproof system, the emphasis should be on fortifying existing systems against known vulnerabilities.
The Increasing Need for AI Regulation
Future safeguards might not solely rely on textual analysis. Feizi hints at the integration of secondary verification tools, such as phone number authentication linked to content submissions or behavioral pattern analysis.
These additional layers could improve defenses against false AI detection and inherent biases.
While AI might be covered with uncertainties, Feizi and Huang are emphatic about the need for an open dialogue on the ethical utilization of LLMs. There is a collective consensus that these tools, if harnessed responsibly, could significantly benefit society, especially in education and countering misinformation.
Read more: These Three Billionaires Are Bullish on Artificial Intelligence, Bearish on Crypto
Trust in Big Tech for AI Governance. Source: Statista
However, the journey ahead is not devoid of challenges. Huang accentuates the importance of establishing foundational ground rules through discussions with policymakers.
A top-down approach, Huang argues, is pivotal for ensuring a coherent framework governing LLMs as the research community relentlessly pursues better detectors and watermarks to curb AI misuse.
Disclaimer
Following the Trust Project guidelines, this feature article presents opinions and perspectives from industry experts or individuals. BeInCrypto is dedicated to transparent reporting, but the views expressed in this article do not necessarily reflect those of BeInCrypto or its staff. Readers should verify information independently and consult with a professional before making decisions based on this content.
Source link